
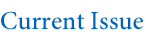
Volume 33, Number 2 (2024)
Computational Foundations for the Second Law of Thermodynamics
Stephen Wolfram
A fundamental explanation for the Second Law is given in terms of the interplay between the phenomenon of computational irreducibility in underlying processes and the computational boundedness of us as observers. This explanation appears to significantly clarify the character and origin of the Second Law and to resolve core aspects of it that were previously mysterious. The approach used allows the Second Law to be broadly generalized beyond its traditional framing in terms of the dynamics of heat—though the approach also suggests limitations to the Second Law, notably in connection with what is termed here the mechanoidal phase. Drawing on some of our other recent work, it is suggested that all three major theories of twentieth-century physics—general relativity, quantum mechanics and statistical mechanics—are ultimately analogous in their foundations and are all derivable from the interplay between computational irreducibility and our characteristics as observers.
Keywords: thermodynamics; second law of thermodynamics; entropy; computational irreducibility; general relativity; quantum mechanics; statistical mechanics; observers; randomness; causality; ruliad; cellular automata
Cite this publication as:
S. Wolfram, “Computational Foundations for the Second Law of Thermodynamics,” Complex Systems, 33(2), 2024 pp. 133–252.
https://doi.org/10.25088/ComplexSystems.33.2.133
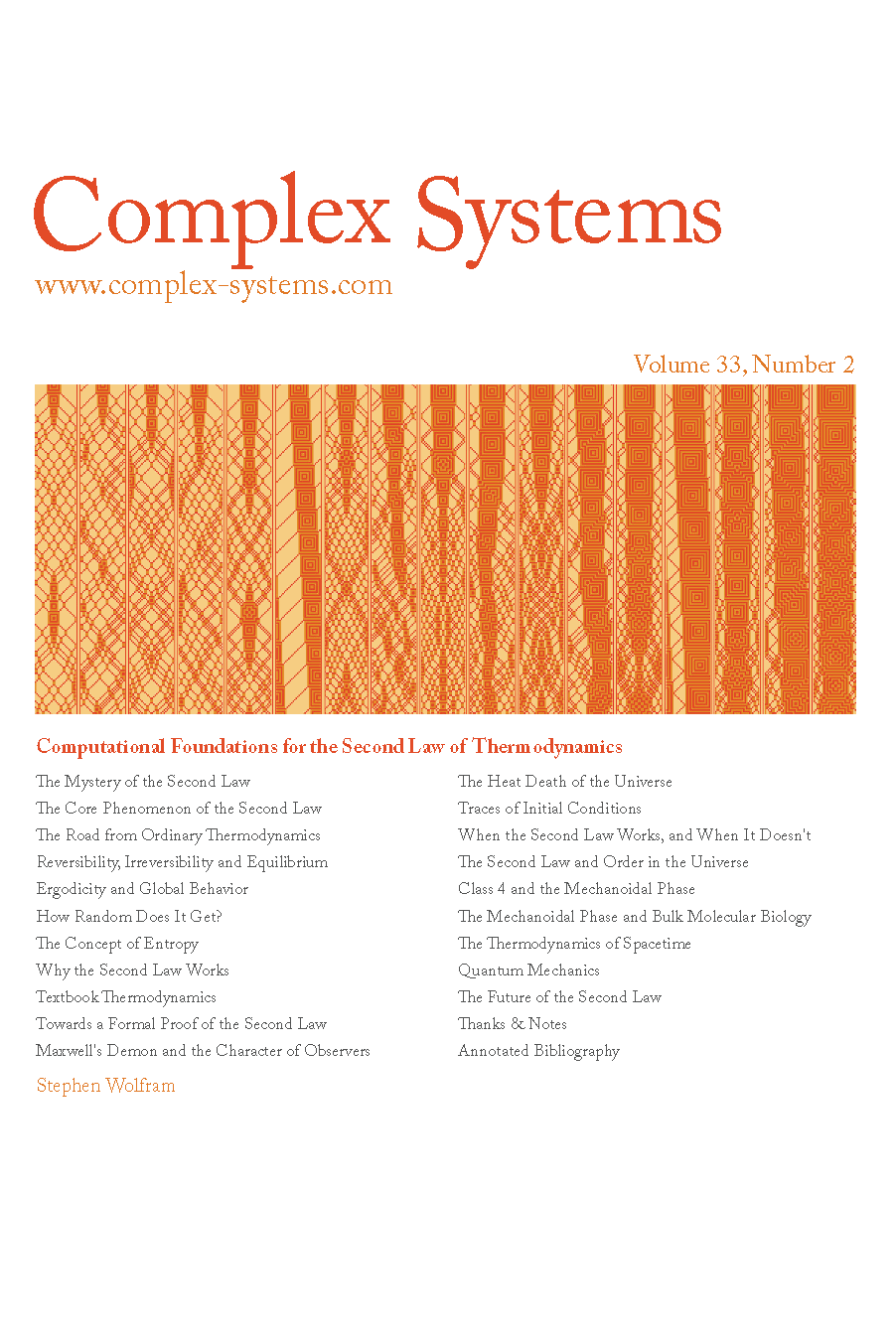

Complex Systems ISSN 0891-2513
© 1987–2024
Complex Systems Publication, Inc.
Published four times annually
Complex Systems Publications, Inc.
P.O. Box 6149
Champaign, IL 61826 USA

Join the leading edge of complex systems research today. There are no publication charges. Authors are provided with 25 reprints of papers published in Complex Systems. Papers may be submitted via the web or email. Find out more »